American football is one of the most popular sports in the world. Our detailed betting guide will show you how to improve your American football betting.
How To Bet On American Football
American football, both the professional and college variety, is the dominant sport in the United States, with its popularity far exceeding baseball, basketball and ice hockey.
The professional form of the sport, the NFL, consists of 32 teams, divided into 8 divisions, with each team playing 16 games through the regular season, 8 at home and 8 away. This makes the season short in time-span in comparison to most major sports leagues, being played from early September through to early February.
The brevity of the NFL season can be a challenge for those of us who enjoy betting on the league, since the limited number of games and opponents can make assessing a team’s true potential difficult. But it’s a challenge that everyone faces, including bookmakers, that can in turn become an advantage for those who are prepared.
Betting on the college version of American football presents similar challenges, with teams playing only a dozen games in a given college football season. But while there are only 32 NFL teams, first division NCAA football consists of over 100 teams including famous footballing colleges USC, Michigan, Ohio State, Alabama, Notre Dame, Oklahoma, Nebraska and Florida State just to name a few.
How To Bet On American Football Betting Guide
Is it easy to bet on the NFL or college football? The answer is no. Just like betting on any other sport or sports league, understanding how to bet on the American football takes focus, persistence and the development of hard earned value betting intuition.
This video is age-restricted. Make sure you are logged into your YouTube account before playing.
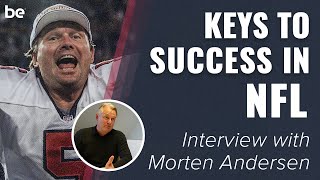
See all bettingexpert American Football betting tips
American Football Odds Explained
American football betting odds come in three popular formats. These are:
- Decimal odds
- Fractional odds
- Moneyline odds
While American sports fans are more familiar with American money line odds, for the purposes of this article, we will deal primarily with decimal odds as they are much easier to work with in terms of basic betting theory and calculations.
Understanding betting odds is key to successful American football betting. Being able to understand the probabilities expressed in betting odds allows you to easily assess betting value. If you cannot determine betting value, then you’re very unlikely to be a long term American football bettor.
The video below provides a good introduction to betting odds and their implied probabilities.
This video is age-restricted. Make sure you are logged into your YouTube account before playing.
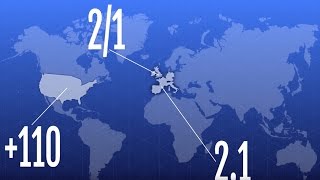
American Football Bet Types
Let’s now discuss how to bet on American football and the range of betting markets available. There are many ways to bet on American football. Bookmakers across the planet now offer a deep menu of American football betting odds, from the more traditional to the more exotic. In this section we will discuss the most popular American football odds,
How To Bet On American Football Lines
The most popular and certainly the most traditional way to bet on American football games is known as the Point Spread.
American football Point Spreads (also known as betting lines or handicaps) is an estimated final margin for a given American football game. Bookmakers determine the margin as a 50/50 bet. In other words, they believe there is a 50% chance the result will end one side of the estimated margin and a 50% chance the result will end on the other side of the estimated margin. Bettors can bet on which side of the margin the game will end on.
American Football Point Spread Explained
So how to bet on American football spreads and handicaps. Let’s consider an example. Let’s say that the Dallas Cowboys are playing the Washington Redskins. The point spread for this game is listed as at bookmaker Ladbrokes:
What does this mean?
Well, for starters the Cowboys are considered the favourites as they are the minus handicap while the Redskins are the underdogs with the plus handicap.
The bookmakers have therefore determined that the final margin will be either side of Dallas by 6.5 points. Bettors can then bet on either side of this margin.
So if you believe Dallas will win by more than 6.5 points (I.e 7 points or more) you bet on Dallas -6.5 points.
Calculating A Winning American Football Spread Bet
On the other hand, if you believe that the Redskins will win the game or at the very least will not lose by more than 6.5 points (6 points or less), you bet on Washington +6.5 points.
Calculating whether your NFL point spread bet was a winner is simple. Let’s say that Dallas wins the game 30 to Washington 20.
If you bet on the favourite, in our example Dallas -6.5, you deduct 6.5 points from their final score. In this case that would leave us with an adjusted final score of Dallas 23.5 Washington 20. With the handicap deducted, Dallas are still winners meaning your bet on Dallas -6.5 points is a winning bet.
On the other hand if you had bet on Washington +6.5 points, we add 6.5 points to their final score, giving us an adjusted final score of Dallas 30 Washington 26.5. Unfortunately for us, even with the handicap added to Washington’s score, Dallas still wins the game so our bet on Washington +6.5 points is a losing bet.
When a team wins a point spread bet, it is often referred to as ‘covering the spread’.
American Football Spread Odds
Odds for American football point spreads range between 1.90 and 1.95 depending upon your bookmaker. If you intend to focus on American football point spreads, we suggest your bookmaker offers at the very least odds of 1.92 on American football point spreads.
If you bet on American football point spreads randomly, over a large enough sample size you will have a 50% strike rate. The table below shows the strike rate needed to make a profit relative to the odds available.
Line Betting Win % Needed To Make Profit
Odds | Win % Required To Profit | Win % Required For 5% ROI |
---|---|---|
1.90 | 52.69% | 55.26% |
1.91 | 52.36% | 54.97% |
1.92 | 52.08% | 54.69% |
1.93 | 51.81% | 54.40% |
1.94 | 51.55% | 54.12% |
1.95 | 51.29% | 53.85% |
Most serious American football bettors look to hit a strike rate of 54%. As you can see, the difference between failure and success betting on American football point spreads may seem slight, but those few percentage points make a world of difference.
How To Bet On American Football Over Unders
Another popular American football bet type is the points total market, also known as Over Unders.
What is an Over Under bet?
An Over Under bet is simple. Similar to a point spread, a bookmaker sets a total number of points that they think will be scored in an American football game, with a 50% chance that the game will end with more than that number of points scored and a 50% chance that the game will end with fewer than that many points scored.
For example, the example below shows the Over Under points total at bookmaker Coral for a game between the Arizona Cardinals and the Atlanta Falcons. As we can see, bettors can bet on either side of 50.5 total points scored in the game.
If you bet on Over 50.5 points, you need the two teams to score a total of at least 51 points for your bet to win. On the other hand, if you bet on Under 50.5 points, you need the two teams to score a total of at most 50 points.
As with point spreads, bookmakers offer odds of between 1.90 and 1.95 for American football point total bets.
It’s also possible to bet on individual team points totals. This is similar to the game total but instead of considering the total combined points scored, all you are concerned with is the points total of a particular team.
How To Bet On American Football Moneylines
American football moneyline bets are simply head to head bets where you are betting on the final result (including over time). No handicaps, no points totals. All that matters is who wins.
Below is an example of an American football moneyline market with odds listed at bookmaker William Hill for a game between the Cleveland Browns and the New York Giants.
As we can see, the Giants are clear favourites in this game at odds of 1.34 while the Browns are at odds of 3.35.
As we said, there are no handicaps involved in American football moneyline bets. You are simply betting on who you think will win the game. If you believe there is value with New York, you bet on them to win the game at odds of 1.34. On the other hand, if you believe there is value with Cleveland you bet on them to win the game at odds of 3.35.
Many American football bettors like to mix and match moneylines with point spreads. For example, Cleveland’s point spread is +7.5 for this game. Many bettors like to hedge the moneyline with the point spread. For example, they will bet $50 on Cleveland to win the game at odds of 3.35 and £55 on them to at least cover the point spread at odds of 1.91. If Cleveland wins the game, you win both bets. If Cleveland loses but by no more than 7 points, you win your point spread bet making a profit of roughly £50, covering your stake for your moneyline bet.
How To Bet On American Football Futures And Outrights
Not only can you bet on American football games, you can also bet on NFL and College football futures and outrights. Betting on American football futures involves betting on the winners of the following:
- NFL Super Bowl Winner
- NFL Conference Champions
- NFL Division Winners
- NFL Most Valuable Player
- NCAA National Champions
- NCAA Heisman Trophy Winner
Other Popular American Football Odds
Thanks to the explosion in online betting, bookmakers now offer a deep menu of American football odds to bet on. The most popular of these additional markets are:
Additional American Football Game Markets
Alternative Point Spreads |
---|
Winning Margins |
Halftime/Fulltime |
Total Touchdowns Scored |
First Touchdown Scorer |
Anytime Touchdown Scorer |
Team To Score First |
First Scoring Play Type |
First Quarter Winner |
First Half Winner |
You can also bet on a range of statistical markets. These include:
Additional American Football Statistical Markets
Individual player passing yards |
---|
Individual player rushing yards |
Individual player receiving yards |
Longest Field Goal |
Longest Touchdown |
Shortest Touchdown |
Total penalties |
Total turnovers |
How To Bet On American Football: Betting Strategies
American football is one of the most difficult sports in the world to bet on. The brevity of the NFL and NCAA seasons coupled with the level of personnel changes season to season can make determining team potential a tough task.
Despite the challenges of betting on American football, there are still bettors who do enjoy betting long term on both the NFL and NCAA. In this section we will show you how you can improve your chances of being one of the few American football bettors who end the season in with a positive return.
Jump To
1. Bet Only When There Is Value
It may seem like overkill, but we can’t stress the point enough. Successful American football betting comes down to one thing, identifying genuine betting value. It’s as simple as that. If you are betting on the NFL or NCAA with absolutely no consideration for betting value, you’re only going to lose money. And by consideration for betting value, we don’t mean taking five minutes to read the views of mainstream football analysts or sitting in front of the TV nodding along with the opinions of the talking heads.
Learn more about value betting
2. Be Prepared To Learn
A mistake many inexperienced American football bettors make is in confusing an understanding of American football with an understanding of American football betting. Don’t get us wrong. Having a deep understanding of the NFL or NCAA and the game of American football is a great start. But the reality is that it is only the first step to developing a mindset for recognising American football value bets. Understanding the game of football and understanding how to bet on football are two very different things.
It is true that understanding how the NFL or NCAA works and possessing a deep and intricate understanding of how the game is played, places you in a great position to develop an understanding of American football betting. But only in time, with experience in dealing with various NFL and NCAA betting markets will you truly be able to adapt your understanding of the NFL and NCAA to your new found knowledge of the markets that you want to bet on.
3. Understanding American Football vs Understanding American Football Markets
Think of it this way. Understanding the NFL is a little like knowing how to bake a cake. Does knowing how to bake a cake help you understand what that cake would be worth to sell on the market? Most likely not. The point is, it’s one thing to determine that a particular NFL game is likely to be a high scoring contest and it’s another thing entirely to determine the value in the odds being offered on the over under line.
Many bettors come into American football betting with a great deal of bravado. They know it all. They never lose. They’re the boss when it comes to picking NFL and NCAA games against the spread. It’s one thing to have bravado and talk a big game, and it’s another entirely to see positive returns from your American football betting. So be humble. Recognise that you don’t know it all. Be prepared to make mistakes. Be prepared to learn.
4. Start By Betting The Teams You Know
A good place to start is by betting on the teams or divisions you know best. Of course, ultimately we want to be able to cover the entire NFL and most of the NCAA. But if you’re new to American football betting, start out by focusing on the teams and/or divisions you are most familiar with.
Most American football fans follow a particular team and have greater exposure to that team’s fortunes. This typically leads to a greater understanding of that team’s common divisional opponents. While we may want to dive in and start betting on games across the entire NFL and NCAA, it’s a good idea to begin simply by focusing on the team’s your most familiar with.
5. Bet The Markets You Know
We have already discussed the most popular American football betting markets. These are:
- Point spreads (betting lines, handicaps)
- Moneylines (head to head, match winner)
- Point Totals (over unders)
There are many more markets available, but most American football bettors focus on at least one of the markets listed above. Each American football betting market has its own quirks and nuances and as you broaden your understanding of NFL or NCAA betting, you’ll discover that some markets make more sense to you than others. So go with your betting intuition.
The most successful NFL and NCAA bettors typically focus on one, maybe two betting markets. It’s not uncommon for experienced American football bettors to focus only on point totals, while others focus on point spreads. The key to long term betting is in focus and specialising. Discover which American football markets you’re consistently able to identify value in and stick to them.
6. Apply Sound Money Management
While the number one key to long term American football betting success is in identifying value, not far behind is the application of sound money management. What does this involve? Put simply, it involves managing your betting bankroll and knowing much to stake on a single bet.
If you seriously want to bet on the NFL and NCAA, you need to be serious about how you manager your betting bankroll. You need to apply your bankroll and staking strategy consistently. If you’re just betting random amounts based on your ‘gut instinct’, that’s fine, but don’t expect to succeed betting on American football games.
How large should your bankroll be? Ideally it should only be as large as you can afford to lose. Yes, we want to see returns betting on NFL and NCAA games, but even equipped with the most sophisticated value identification and money management strategies, results can often go against us. Losing streaks a fact of life for even the most successful American football bettors. The only way to earn consistent returns while protecting yourself against losing streaks, is in the application of a sound money management strategy.
Learn more about bankroll management
7. Keep A Record Of Your Betting
Every serious bettor, whether they bet on the NFL, NCAA football or any other league, keeps a record of their bets. And if you’re not keeping a record of your bets, then do not expect to learn, do not expect to improve your returns.
Your record of American football betting should include the following:
- Date
- Bet Type (market)
- Bet Details
- Bookmaker
- Your Stake
- Your Odds
- Your Profit/Loss
- Comments/Notes
If you’re not keeping these kinds of detailed records of your NFL and NCAA betting, then you are more than likely going to lose money long term. Why? Because reviewing your bet history is key to improving your future betting returns. By keeping records you will see that you are more profitable betting on particular American football markets. Likewise you will be able to see where you did not maintain your discipline and apply a consistent staking strategy. In short, keeping records of your American football betting will make you increasingly self aware of your betting performance and tendencies.
8. Assess Team Performance Accurately
This is where the rubber meets the road. Identifying betting value comes down to your ability to identify team performance.
Unfortunately, this is not an easy thing to do. Bookmakers have some of the sharpest analytical tools and minds at their disposal, framing NFL and NCAA odds that are far more often than not, incredibly accurate. Fortunately, for American football bettors, the bookmakers don’t always get it right. In fact each season there are one, two maybe even three NFL teams that the bookmakers underestimate. Likewise there are teams bookmakers overestimate. It’s your task as an American football bettor, to identify those teams the bookmakers have incorrectly assessed.
How can we do this? There are a number of things to consider when determining team performance and potential in the NFL and NCAA football.
Home Field Advantage In The NFL
The topic of home vs away form is open for debate. Many believe that certain teams have a fixed home field advantage (teams such as Seattle, New Orleans, Denver) while others argue that while some teams may exhibit an enhanced home field advantage over a small sample size of games (perhaps as long as two or three seasons), home field advantage in the NFL is consistent long term with no team truly having a greater home field advantage than any other.
Home field advantage in the NFL is considered to be worth 3 points. What does this mean? Well, if two teams are considered equal in potential, the home team is considered to be 3 points better purely because they are playing at home. This amounts to a six point swing between home and away performance.
For example, let’s consider a game between the New York Jets and the Denver Broncos with the game being played in Denver. On a neutral field, we consider Denver to be a 4 point better team than the Jets. If the game then is played in Denver, we add 3 points for home field advantage to Denver making them a 7 point better team than the Jets. If the game is played in New York however, we add 3 points for home field advantage to the Jets, making Denver only a 1 point better team with the game played on the Jets home field. All things considered, there is a 6 point swing between a team playing at home as opposed to playing away.
The most common explanations for why home field advantage exists in the NFL are crowd noise (which impacts the performance of the away team’s offence), officiating (with referees favouring home teams, displaying all kinds of very human psychological biases) and the weather (warm weather teams generally dislike playing in cold weather venues).
Injuries and Roster Additions/Subtractions
Many experienced American football bettors take advantage of early season odds by accurately assessing the impact of each team’s roster additions and subtractions over the off season. For example, while the addition of a high profile wide receiver may sound exciting to fans and TV pundits, the loss of two key offensive lineman may have a greater impact on a team’s fortunes causing them to perform well below expectations of both fans and bookmakers. Being able to accurately assess the potential of a given NFL or NCAA roster early in the season, can provide you with a huge advantage over the bookmakers for a number of weeks.
Being able to correctly assess the impact of injuries is another way savvy American football bettors take advantage of NFL and NCAA odds. They may not be household names, but injuries to both offensive and defensive linemen can have a huge impact on a team’s fortunes. Likewise, if a team is down to their third or fourth cornerback due to a spate of injuries to that position, don’t expect the defence to keep the opposing team’s passing attack under control. Yes, an injury to a team’s quarterback or star running back or hall of fame bound wide receiver may get the headlines, but if you want to bet on American football games, evaluate each team’s depth chart. You’ll be surprised how often guys you’ve never heard of seriously impact a team’s performance.
Tactical Matchups
There’s the old saying in boxing that styles make fights. This is certainly true when it comes to the NFL and indeed NCAA football. How often do we see teams of inferior talent defeat teams we consider to be far more gifted? This is particularly true in divisional matchups where the team’s know each other intimately.
The reality is that while some teams may be inadequate in certain areas, when it comes to matching up against a more talented opponent, they have precisely the pieces required to trouble the highly favoured rival. This is where your understanding of the game of football can provide you with enough insight to identify betting value. Understanding how teams matchup, where their strengths and weaknesses lie can be a great way of finding NCAA and NFL value bets.
Develop An American Football Betting Model
Many successful NFL and NCAA football bettors have earned their living by developing sophisticated statistical models allowing them to analyse and assess NFL and NCAA team performance and potential more accurately than bookmaker American football odds suggest.
Developing an NFL or NCAA betting model or system takes time and dedication. But the rewards can be huge. Some American football betting models only take point differential into account while others look deeply into and a range game data and statistical categories. Most American football betting models consider at least one of or a number of the following:
- Points For/Against
- Total Yards For/Against
- Turnovers For/Against
- Yards per Rush For/Against
- Yard per Pass For/Against
- Yard per Play For/Against
Regardless of what statistics you want to apply to your model, the aim is the same as any other, and that is to determine each team’s potential and in turn, the probability of them winning a given game more accurately than bookmakers do.
It’s also worth noting that while it would be great to develop an NCAA or NFL betting model that identifies value bets in every game, the reality is that any model is only going to discover a value betting opportunity in one, two perhaps three games each week of the season.
9. Bet Against Favourites
As we’ve stated relentlessly in this article, the key to long term betting success in American football is in identifying value betting opportunities. Sure, a team may be in horrible form and are coming up a highly fancied opponent, but while their chances of winning the game by our own estimation are slight, the fact that we believe they are a better chance to win the game than what the long odds would suggest makes them a value bet regardless of how slight those chances may be. It’s in consistently betting on instances such as these that will make you a successful NFL or NCAA bettor.
When it comes to American football betting, there are two primary market biases. These are:
- Higher than average point totals
- Short odds home favourites
Let’s start with points totals. Most football fans like to see high scoring games and as a result, the majority of American football bettors will bet Over in the points total market. Your average casual bettor doesn’t care too much about thorough analysis. He bets with his gut instinct.
When it comes to point totals, the majority of casual bettors subconsciously bet with aggression and as a result want to see points scored. The weight of this casual money on the Over distorts the market making the odds for Over shorter than they should be, while the odds for the Under swell excessively, meaning that more often than not, betting on the Under is the value bet.
Similarly, most casual bettors look for a lock. They don’t want to bet on teams at long odds. They want a winner this week. As result many casual bettors bet heavily on home NFL and NCAA favourites, sometimes at very short odds. Again, this weight of casual money distorts the market making the home favourite far shorter than what they should be, often seeing the long odds road underdog the value bet.
These are two simple American football betting market biases that can be exploited to your advantage. No, betting the Under on every NFL or NCAA game or betting on every long odds road dog is not going to make you money. But it’s important to keep these biases in mind when preparing your weekly best bets.
10. Follow American Football Tipsters
The issue is of course, how do you find NCAA and NFL tipsters that you can count on? This is perhaps more difficult with American football than any other sport. Just search NFL picks on Google and you’ll find an endless stream of websites and prognosticators claiming to offer reliable NFL tips, with many asking high subscription fees for their selections.
We strongly recommend that you do not pay for NCAA and NFL tipsters picks. Regardless of the results tipsters may be boasting, it’s far too common for these to be falsified records. Following these so called experts, will only cost you subscription fees not to mention money lost at your bookmaker.
The good news is that there is no need to pay for reliable NFL and NCAA selections. At bettingexpert, we have a number of successful American football tipsters, each posting their selections throughout the season. And yes, their tips are absolutely free. Every tipster’s record is clear to view so you can decided which tipsters you want to follow and those you don’t.
Follow Our NFL Tipsters
11. Have Multiple Bookmaker Accounts For Betting
While having just one bookmaker for your American football betting may be convenient, it’s not going to help you be a winning NFL or NCAA bettor long term.
We recommend that you have at least three accounts for your American football betting. This will give you enough flexibility so that you can take the best odds on offer for whichever team or outcome you are planning on betting on.
Sure, the difference between betting on a team at odds of 2.10 may not seem so different from odds on that same team at another bookmaker of 2.20, but over the long term it all adds up and if you’re serious about your NFL or NCAA betting, it can add up, simply by taking the marginally better odds on offer.
Where Can I Bet On NFL Or NCAA Football Games?
Every bookmaker on the planet offers odds on NFL or NCAA games. For betting on American football games, we recommend the following bookmakers. Click the logo to read about their current new customer bonus offer.
Detailed Bookmaker Reviews
To find the best bookmakers for you NFL betting, please refer to our list of detailed bookmaker reviews.
How The NFL Works
The National Football League (NFL) is America’s elite football league. Comprised of 32 teams, the NFL season is played over 17 week regular season with each team playing 16 regular season games with one bye week.
NFL Conferences & Divisions
The 32 NFL teams are divided into two 16 team conferences, the National Football Conference (NFC) and the American Football Conference (AFC). Each conference is comprised of four divisions each consisting of four teams. Each division is based on a geographical region – East, West, North And South – with teams generally aligned by their location (although peculiarities exist such as Dallas designated to the east and Indianapolis to the south).
National Football Conference Divisions
NFC East | NFC North | NFC South | NFC West |
---|---|---|---|
Dallas Cowboys | Chicago Bears | Atlanta Falcons | Arizona Cardinals |
New York Giants | Detroit Lions | Carolina Panthers | Los Angeles Rams |
Philadelphia Eagles | Green Bay Packers | New Orleans Saints | San Francisco 49ers |
Washington Redskins | Minnesota Vikings | Tampa Bay Bucs | Seattle Seahawks |
American Football Conference Divisions
AFC East | AFC North | AFC South | AFC West |
---|---|---|---|
Buffalo Bills | Baltimore Ravens | Houston Texans | Denver Broncos |
Miami Dolphins | Cincinnati Bengals | Indianapolis Colts | Kansas City Chiefs |
New England Patriots | Cleveland Browns | Jacksonville Jaguars | Oakland Raiders |
New York Jets | Pittsburgh Steelers | Tennessee Titans | San Diego Chargers |
How The NFL Schedule Is Determined
Each team plays the other three teams in their division twice during the regular season, once at home and once away.
Eight games on the schedule are comprised of four games against another division within the conference and four games against a division outside of the conference. These eight games are based on a four year rotation.
The final two games on the schedule are against conference opponents who finished in the same place in their division the previous season but who the team is not already scheduled to play. As each team plays another division in their conference, these final two games are against teams from the other two divisions.
Composition Of NFL Team Schedule
Opponents | Games |
---|---|
Division Opponents at home | 3 |
Division Opponents away | 3 |
Other Division within Conference at home | 2 |
Other Division within Conference away | 2 |
Other Division out of Conference at home | 2 |
Other Division out of Conference away | 2 |
Conference opponent by position at home | 1 |
Conference opponent by position away | 1 |
This video is age-restricted. Make sure you are logged into your YouTube account before playing.
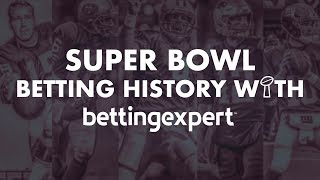
How The NFL Playoffs Work
Six teams from each conference advance to the playoffs that begin in early January. These six teams feature each of the four division winners with two ‘wildcards’ also advancing to the playoffs. The two wild card teams are those which posted the best win/loss records in their conference but did not win their division.
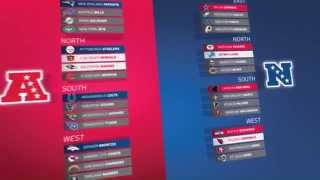
The six advancing teams are ranked firstly in order of win/loss record by division winner and then win/loss record of wild card team. This means that the top four seeds are each division winners with the fifth and sixth seeds taken by the two wildcard teams.
NFL Wildcard Round
The top two seeds in each conference do not play the first week of the playoffs, with the 3rd seed hosting the 6th seed and the 4th seed hosting the 5th seed. The winners of these games then progress to the Divisional Round, with 1st seed hosting the lowest seeded winner of the Wildcard Round and the 2nd seed hosting the other Wildcard winner.
NFL Divisional Round
The winners of the Divisional Round progress to the Conference Championship game, with the highest seeded team of the two earning the right to host the Championship game on their home field.
NFL Conference Championship Games And The Super Bowl
The two winners of the Conference Championships advance to the Super Bowl played in early February.
Example: NFL Playoffs 2015/16
Below we can see the AFC Playoff Bracket for the 2015/16 NFL season with the Denver Broncos winning the conference and representing the AFC at the Super Bowl.
Below we can see the NFC Playoff Bracket for the 2015/16 NFL season with the Carolina Panthers winning their way to the Super Bowl to face the AFC Champion Denver Broncos.