How To Bet On Football
Betting On Football is a world of its own. Here we will discuss the most popular football match betting markets.
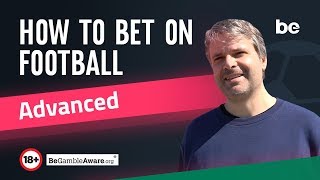
Betting On Football – Fulltime Result
The most popular form of betting on football is fulltime result, with two betting types being the most common – 1X2 (Win/Draw/Lose) and Asian Handicap. With 1X2 betting, you can bet on either team to win or for the match to end in a draw. This form of betting is often referred to as 3-way betting. Asian Handicap on the other hand, was essentially created to eliminate the draw as an option and to also make seemingly one-sided matches a little more interesting. Bookmakers will offer you a goal handicap that is added to one team’s fulltime score or deducted from the other.
For example, a handicap of +1.5 goals, means that if you add 1.5 goals to the fulltime score of that team, and the score is then greater than their opposition, that bet is then a winning bet. That team has ‘covered the handicap’. A handicap of -1.5 goals on the other hand is deducted from that team. However, if that team’s score remains greater than their opponents once the handicap is deducted, that is a winning bet. It’s also worth noting that the regular length of a football match is 90 minutes. In many matches, it’s not uncommon to see two or three minutes of added injury time, but it still counts as normal time for betting purposes. Extra time or penalty shootout do not count in normal 3-way bets.
Match Result 1X2 Odds Explained
If you’re asking ‘How can I bet on football teams?‘, the match result is the most common answer. Match result betting is often referred to as 1X2 betting. It’s easy to learn how to read 1X2 odds? The 1 signifies one team (usually the home club), the X signifies the Draw and the 2 signifies the opposing team (usually the away club). Match result is the most common form of football betting (along with Asian handicaps).
How to read 1X2 odds? Well we can see that Burnley are the home team (1) at odds of 2.50, the draw (X) is at odds of 3.25 while Swansea are the away team(2) at odds of 2.80
It’s important to note, that when betting in knockout tournaments, there is often the potential for extra time to be played. If this is the case, 1X2 betting only covers the regulation 90 minutes. If you have bet on the match to end in a draw, then your bet is a winner even though extra time will be played. If you have bet on either team to win and the game goes into extra time, your bet is lost.
Asian Handicap Odds Explained
Another popular way of betting on football is the Asian Handicap (AH) market. In recent years the popularity of Asian handicap betting has exploded with tens of thousands of pounds being bet on football handicaps every weekend. What does Asian Handicap mean? To the uninitiated, Asian Handicap odds can appear a little complicated at first glance. The video below provides a basic introduction:
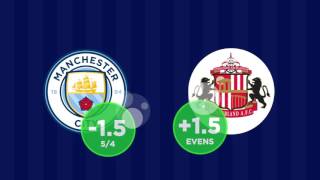
To put it simply, Asian Handicaps give both teams a goals handicap, either a goals advantage (+) or a goals deficit (-),.
Below we can see an example of an Asian Handicap odds, with odds at BetVictor for an upcoming match between Arsenal and Liverpool. Arsenal have a handicap of -0.5 goals while Liverpool have a handicap of +0.5 goals. What does this mean? It means that if you bet on Arsenal -0.5 goals, Arsenal must win the match for you to win your bet. On the other hand, if you bet on Liverpool, then either a Liverpool win or a draw will be enough for you to win your bet.
The easiest way to understand handicap betting is to take the full time result and adjust the scoreline to incorporate each team’s handicap. If a team has a minus handicap (as Arsenal does in the above example) we deduct that handicap from their fulltime score. If they still lead after the handicap has been deducted, then that is a winning handicap bet.
On the other hand, if a team has a positive handicap (as Liverpool does), then we simply add the handicap to their fulltime score. If they then lead after the handicap has been added, then that is the winning handicap bet.
Calculating Asian Handicaps: Two Examples
So let’s say you have bet on Arsenal with the -0.5 handicap. The fulltime result is Arsenal 2, Liverpool 1. Is your handicap bet on Arsenal a winner? We simply deduct Arsenal’s -0.5 goal handicap and we have an adjusted scoreline of Arsenal 1.5, Liverpool 1. With their -0.5 goal handicap deducted, Arsenal still “win” the match by half a goal. In this case, your handicap bet on Arsenal is a winner.
But what if we bet on Liverpool with the +0.5 goal handicap? In this example, let’s then say that the match ended in a draw 2-2. Is our Liverpool handicap bet a winner? We add the +0.5 goal handicap to Liverpool’s score, giving us an adjusted scoreline of Arsenal 2, Liverpool 2.5. With this adjusted scoreline, Liverpool “wins”, meaning our handicap bet on Liverpool is a winning wager.
Asian handicap betting is a great way to bet on football, making even the most lopsided contest suddenly a thrilling match, not to mention the potential to find hidden value in Asian Handicap football betting markets.
There is also the possibility of betting on what are known as Quarter Asian Handicaps.
Double Chance Odds Explained
A Double Chance bet allows you to bet on two out of the three possible outcomes of a football match in one bet. In a Double Chance bet, you can bet on the following fulltime result combinations:
- Home Win / Draw
- Away Win / Draw
- Home Win / Away Win
Below we can see an example of a Double Chance betting market, with odds at Paddy Power for an upcoming match between Manchester City and Borussia Dortmund.
As with the Draw No Bet market, the odds offered on Double Chance are typically far lower than if you were to bet on just one result, as with Double Chance your bet is covering a combination of two possible results.
Correct Score Odds Explained
A Correct Score bet is betting on the exact fulltime scoreline of a given football match. These bets are popular with casual bettors as the odds on any selection are usually long.
A popular Correct Score betting strategy is to bet on a combination Correct Scores. This is often referred to as ‘dutching’. For example, if you think a particular match is going to finish 2-0 to the home side, you can pick this as your Correct Score bet. But you can also cover a range of potential scorelines, not just the 2-0. You could for instance place additional bets on 1-0, 1-1, 2-1. Your overall returns will be shorter but in covering a range of Correct Scores, you’re providing yourself with some insurance should your initial bet of 2-0 fail.
Betting On Football – Half Time Full Time
Instead of combining the first and second half, you can bet on the outcome of just the first half. Especially if you spot matches where teams are close or if they are known for great defense, you can find great value in knowing these tendencies. It’s also a nice option to play outsiders here that dig in, often they manage to hold the game to a draw until halftime. Betting on the result after both half and full time is also popular, but quite difficult. The basic notion is the same as with 1×2, but in this case you bet on the outcome after each half and you have to get both parts of the bet correct in order to win.
To succeed at this type of betting on football, it’s important to have a sense for how a match may play out and the tempo of both competiting teams. A team may be certain of victory against an inferior opponent but they may also be a team that is prone to slow starts in such situations. In this case a HT/FT bet of Draw/Win could be great value.
Halftime Fulltime Odds Explained
Below we can see an example of a Halftime / Fulltime betting market, with odds listed at Paddy Power:
In this example, the German club Hertha Berlin are hosting the Danish club Brondby. What makes the Halftime / Fulltime market so intriguing is that it doesn’t simply involve picking the fulltime result, but rather involves predicting how the match will playout across both halves. For example, we may believe that Hertha Berlin will win, but we may also believe they will get off to a slow start. So we will back a halftime Draw with Hertha leading at fulltime. We may even believe Brondby will lead at halftime with Hertha coming on strong in the second half to snatch the victory, in which case we would bet on a Brondby / Hertha result at odds of 23.00.
In this way Halftime / Fulltime betting can prove to be a great way to bet, particularly if you possess an intimate knowledge of both a team’s tactical approach and psychological tendencies.
Accumulators / Combo bets / Parlay bets
Essentially, these are three different names for the same thing: Placing a bet on more than just one outcome. Putting a number of selections on one betting slip can give the promise of great returns as each of the odds multipy into the next.
For example, the odds on a combo bet of 4 selections is calculated the following way:
Selection One Odds 2.00 |
Selection Two Odds 1.50 |
Selection Three Odds 2.35 |
Selection Four Odds 1.35. |
= 2.00 x 1.50 x 2.35 x 1.35 |
= Combo Odds of 9.52. |
Football accumulators are always a popular betting option when it comes to football. Throughout the season, millions of pounds are wagered on football ‘accas’, with punters attracted by the promise of huge payouts for small stakes.
A word of caution when playing combo bets. Unless you have found value in each of your selections, then the bookmaker commission is multiplied with each selection diminishing the value of your combo bet. However, if you have found value in each of your selections, then that value is multiplied into each selection you make.
Predicting the Number of Goals and Exact Score
When betting on football, you can also bet on the total number of goals or the fulltime score. Odds on these kinds of bets are often rather high but are rarely worthwhile bets. Outcomes such as these are hard to predict and precision is needed in this kind of betting. On top of a low strike rate that makes for long losing runs, bookies also tend to have very large margins on these kind of markets for the simple reason people don’t notice the lower odds as much on a market with this many outcomes. These margins are also put in place by bookmakers because of the difficulty in predicting these results. In this way, bookmakers are essentially protecting themselves from the randomness of outcome. Odds on other soccer markets simply tend to be more competitive, consistent and as a result, worthy of investing your time and money in.
Over/Under bets – Goal Totals
Another common bet is to predict if the total number of goals precedes a predefined number. Often 2.5 goals is set as a common benchmark. You simply bet on whether a match will end with fewer than 2.5 goals or more than 2.5 goals. In other words, will the match end with 3 or more goals or fewer than 3 goals.
Over Under Odds Explained
Betting on goals over/under is another very popular form of football betting. Over Under betting doesn’t require you to pick the result of a particular game, but rather to determine whether a game will have over or under a certain number of goals. The standard bet is 2.5 goals, though some bookmakers will offer anywhere from the 0.5 goal line up to 7.5 goals.
Below we can see an example of an Over Under 2.5 goals betting market, with odds at Paddy Power for an upcoming match.
As you can see, Over Under betting is one of the simplest forms of betting. If you bet on Over 2.5 goals, then so long as there are a minimum of 3 goals scored in the match, you’re a winner. If the match ends with 2 goals or fewer, then your Over 2.5 goals bet is lost. If you bet on Under 2.5 goals, then your bet is a winner so long as there are a maximum of 2 goals scored in the match. Any more and your bet is lost.
The table below shows the winning results based on basic Over Under goal total lines and the total number of goals scored in a match.
Over Under | 0 Goals | 1 Goal | 2 Goals | 3 Goals | 4 Goals | 5 Goals | 6 Goals |
---|---|---|---|---|---|---|---|
0.5 goals | Under | Over | Over | Over | Over | Over | Over |
1.5 goals | Under | Under | Over | Over | Over | Over | Over |
2.5 goals | Under | Under | Under | Over | Over | Over | Over |
3.5 goals | Under | Under | Under | Under | Over | Over | Over |
4.5 goals | Under | Under | Under | Under | Under | Over | Over |
Full Goal Over Under Lines
There are also Full Over Under lines. An example would be Over Under 3 goals. If you bet on the Over, then you require at least 4 goals to be scored in the match for your bet to win. If you bet on the Under, then you require a maximum of just 2 goals to be scored for your bet to win. If the match ends with exactly 3 goals scored, your bet will be voided and your stake refunded.
The table below shows the winning results based on full goal Over/Under lines and the number of goals scored in a match.
Over Under | 0 Goals | 1 Goal | 2 Goals | 3 Goals | 4 Goals | 5 Goals | 6 Goals |
---|---|---|---|---|---|---|---|
1 goal | Under | Refund | Over | Over | Over | Over | Over |
2 goals | Under | Under | Refund | Over | Over | Over | Over |
3 goals | Under | Under | Under | Refund | Over | Over | Over |
4 goals | Under | Under | Under | Under | Refund | Over | Over |
5 goals | Under | Under | Under | Under | Under | Refund | Over |
Quarter Over Under Lines
Although not as common as Asian Handicaps, there are also Quarter Over/Under lines. An example would be a line of Over Under 2.75 goals. Just like Quarter Asian Handicaps, this can be consider a ‘split bet’. So if you bet on Over 2.75 goals, your bet is split into two halves, with half of your stake on Over 2.5 goals, and the other half stake on Over 3 goals (and conversely if you bet on Under 2.75 goals). In this instance, if you bet on the Over 2.75 goals, then so long as there are 4 goals scored, your bet is a winner. If the match ends with 3 goals scored, you will win your Over 2.5 goals half bet with the other half bet on Over 3 goals being refunded. If you had bet on Under 2.75 goals, then so long as there are just 2 goals or fewer, your bet wins. If there are 3 goals scored, you would lose your Under 2.5 half bet, while your Under 3 goals half bet would be refunded.
Betting On Football – Draw No Bet
In this bet, a draw is not an option, meaning that in the case of a draw, you get your stake back. As a consequence, the odds are lower on each team to win than in traditional 3-way betting. This is just the same bet as an Asian Handicap +0 bet on any given team.
See all football betting tips posted on bettingexpert at the moment
Draw No Bet simply means you place a bet on one team to win the match and if the game finishes as a draw you will have your stake refunded (i.e there is no bet).
It’s a fairly simple bet as you can see. You can bet on either Chelsea or West Ham to win the match, with your stake protected should the match end in a draw. With the potential for a draw eliminated from the market, DNB odds are lower than if you bet on either team in the 1X2 match result market.
Betting On Football Goalscorer
Here, you are betting on which player will score the first goal. Pretty simple, right? It is a fairly simple concept but take time to check the rules that apply at your bookie. Issues like own goal and substitutions can differ from place to place. In some cases, your player doesn’t need to be the first to score. Some bookmakers offer a variation of the bet where you also win as long as your player scores the second or third goal. These bets are called Each Way First Goal Scorer. Yet another variation is called Last Goal Scorer. Here you bet on who will score the last goal. Alternatively, you can bet on a player to score at any time during the match. This is known as Scorer Anytime. Betting on first scorer and the final result is also a possibility and is called Scorecast. But not one to be recommended! Bookmakers are big time favourites to win that one so use your money on something more fun and with consistency.
First Goalscorer Odds Explained
First Goalscorer betting simply involves picking a player and betting on them to score the first goal of a given match. Below we can see an example of a First Goalscorer betting market, with odds at BetVictor for an upcoming match:
It’s important to note that if you pick a player to score first and they don’t start the match, i.e. they’re a substitute, your stake will be refunded if a goal is scored before they enter the pitch. It’s also worth noting that you can actually bet on No Goalscorer, which is essentially the same as betting on a 0-0 draw.
Anytime Goalscorer Odds Explained
Similar to First Goalscorer betting, Anytime Goalscorer betting involves picking a player to score at any stage of a given match, regardless of whether a goal has been scored already. Below we can see an example of an Anytime Goalscorer betting market, with odds at BetVictor for an upcoming match:
The odds are typically shorter than First Goalscorer betting as with Anytime Goalscorer it does not matter when your player scores, just so long as he does score.
League/Tournament Top Goalscorer Odds Explained
An always popular market is the League or Tournament Top Goalscorer. This is simply a matter of betting on the player who you think will finish the league season or tournament as the top goalscorer.
As with most outright markets, it is possible to place bets throughout the season, giving you the potential to trade in and out of the market as well as identify value based on upcoming fixtures, injuries, suspensions and tactical tendencies.
Next Manager & Player Transfer Odds Explained
A slightly perverse but always popular football betting market are the array of markets that come under the heading of manager betting. These markets include betting on the next manager to be sacked in a given league, the next permanent manager to be appointed to a particular club as well as betting on manager of the year awards.Above is an example of the Next Manager market, in this case, odds from BetVictor for the next fulltime Arsenal manager.
Player Transfer Odds Explained
A popular specials market, particularly during the off-season are player and club transfer markets. Transfer betting typically involves betting on which club a player will sign with next and can sometimes involve a timeframe, for example, betting on the club a player will play with next following the summer transfer window.
Above is an example of Next Club odds, in this case odds posted by Power Power for Cristiano Ronaldo’s next permanent club.
Football Value Bets
Let’s first make a distinction. We are not, and should not, be interested in football gambling. We are not interested in ‘gambling’. The term gambling implies a certain reckless degree of risk-taking. And yes, there is risk involved in implementing even the most considered football betting strategy, but was distinguishes football betting from football gambling is that football betting is about identifying value opportunities and exploiting them, while football gambling is merely about having a punt and hoping to win big on the weekend’s football. If you want one piece of football betting advice, it is this- only bet when there is value.
What Is Value Football Betting?
The key to serious football betting (or betting on any other sports for that matter) is in finding what we call value bets. A value bet is when we believe that the chances of a particular outcome are higher than what the odds suggest. It point cannot be understated. You should only bet when there is betting value.
This is why understanding the implied probability reflected in football betting odds is so important to understand. Why is it so important? Let us repeat ourselves to make it clear – we should only bet when we believe the chances of a particular outcome are greater than what the odds on offer reflect.
An Example: Liverpool vs Sunderland
Value football betting is where your knowledge of football comes into play. Let’s consider two Premier League clubs in this example, Liverpool at home to Sunderland. The bookies are offering the following odds for the match result market:
- Liverpool 1.50
- Draw 4.50
- Sunderland 6.50
We are keen Premier League viewers and have undertaken additional research on these two clubs including the possible team line ups for this game, knowing that Liverpool will be without their leading goalscorer and their best defender.
We also know that Sunderland needs a win to avoid relegation and their leading goalscorer will be starting this match after a couple of weeks suspended. We have also taken a close look at some historical football data and have seen that Liverpool tends to underperform when expected to cruise to a comfortable victory at home.
Given all of this information, we believe that Sunderland have a good chance of winning this game, making the odds of 6.50 well worth a bet. These odds suggest that Sunderland are a 15.4% chance to win. We believe their chances, while still slight, are closer to 20%. This is a value bet because we believe the chances of Sunderland winning are greater than those reflected in the odds being offered.
Calculating A Football Value Bet
As discussed, the odds of Sunderland winning the match are 6.50, which means there is a 15.4% implied probability of them winning. But through our knowledge and analysis, we believe Sunderland’s chances are greater than that, we believe that they have a 20% chance of winning.
We calculate betting value with the following formula:
Value | = | (Decimal odds * Our Assessed Probability) – 1 |
So in our example, we have Sunderland at odds of 6.50 and our assessed probability of them winning is 20%. Do we have a value bet?
Value | = | (6.50 * 0.20) – 1 |
Value | = | 1.30 – 1 |
Value | = | +0.30 |
When the calculation provides us with a + figure, we have a value bet. In this case we believe Sunderland are an excellent value bet at +30%. If the calculation returns a figure equal to or below 0, there is no betting value and we should not place a bet.
To be a successful long term football bettor, it’s important to expand your knowledge of both football the game, while likewise expanding your knowledge of football betting markets. Developing both in unison will enhance your ability to recognise football betting value.
How Much Should You Bet?
Now we come to the topic of staking. Yes, some people bet just for a bit of fun and that’s fine, but here we’re interested in serious football betting.
However, regardless of whether you intend to be a serious football bettor or a fan who enjoys a punt on the weekend, there are three crucial things to remember when it comes to your staking:
- only bet what you can afford to lose
- never chase losses
- don’t increase your stakes when on a winning streak or a run of losses
10 Rules Of Betting On Football
Some of you may be wondering if there is a foolproof way to bet on football. Unfortunately, there is no football betting strategy that will guarantee you profits. This simply isn’t possible and anybody telling you that they know how to bet on football without losing or have discovered a football betting system that works 100% of the time, winning football bets with ease, is lying to you. So do not be fooled by football betting sites that claim to have a simple football betting system that will make you rich and certainly do not pay money to sites that claim to show you “how to win big on football bets”. It’s pure marketing spin hoping to seduce you into parting ways with your hard earned money. The reality is that while it would be nice to win football bets every time, but long term betting on football is like betting on any other sport. It takes discipline, experience and persistence. If you’re keen to take up the challenge and seriously learn how to bet on football successfully, you’ve come to the right place. While it’s impossible to win every football bet you place, there are a number of things you can do to be a serious football bettor.
#1 Bet Only When There Is Value
By now you’re probably tired of reading this. But it’s a point that many football punters continually fail to recognise, the best football bets, in fact, the only football bets worth making are those where you have identified genuine betting value. If you place bets without recognising genuine betting value, you are only going to lose money in the long run. It’s as simple as that.
If you skipped over the section on Value Football Betting, please read it now before continuing with our guide.
#2 Be Prepared To Learn
A mistake made by many novice bettors is to believe that because they possess an intimate knowledge of football, this knowledge immediately translates to a nuanced understanding of football betting markets. The reality is that understanding football and understanding football betting are two very different things.
Yes, of course, without an understanding of football, you will not be able to grasp the nuances of football betting, and yes, in time an understanding of both football the game, and football betting will compliment one another. But only in time will you come to appreciate the dynamics of football betting markets and how approaching a football match as a fan differs greatly from the approach of a seasoned football bettor.
The best way to develop this understanding is to begin with the leagues you know best and to keep your bets small. In fact some of the most successful football bettors began their journey by not placing real bets at all, but rather by ‘paper trading’ where you keep a record of fictitious bets so as to gain experience without the risk of losing any real money. These successful bettors only began placing real bets once they had developed a deep understanding of football betting markets.
Humility is a good thing, particularly when it comes to football betting. Sure, you may possess a deeper knowledge of each Premier League club than many media pundits but don’t confuse this knowledge for an understanding of football betting markets.
To learn how to approach a range of football betting markets, leagues and tournaments, please refer to our detailed series of how to bet on football guides.
#3 Bet The Leagues You Know
As stated previously, the best way to develop a genuine understanding of football betting markets is to start with the leagues you know. But even once you have developed an understanding of these markets, remain focused on the leagues your understand best. If there is no action in those leagues this week, then take a break. Or use that time to research other leagues you may want to bet on in the future. But by no means should you go hunting for a bet just for the sake of having a bet. This will only lead to ruin.
Many of the most successful football bettors and the best bettingexpert tipsters focus solely on two, three, maybe four leagues. They study everything there is to know about those leagues and through time develop an understanding that exceeds that of the bookmakers.
Think about it this way, bookmakers have some of the most cutting edge analysis tools at their disposal, tools that incorporate dozens of variables enabling them to set what are for the majority of the time, incredibly accurate odds. Do you really think you’re going to outwit them with half an hour of ‘analysis’ on a league you have at best only a broad understanding of? The answer is clearly no.
#4 Bet The Markets You Know
As discussed above, there are literally dozens of football betting markets, each with their own quirks and nuances. As you develop your understanding of football betting, you’ll find yourself attracted to particular markets more than others. Put simply, some betting markets make more sense to us than others. So go with your intuition and develop a rich understanding of these markets.
Many of the best football bettors focus on one or two markets. There are many successful football bettors who bet only on Asian Handicaps, while others focus on 1X2 match results.
Others look to exploit in-play markets by betting on the team to score first, while others keep their focus solely on first half markets. In fact the quirkier the market, the more your experience will provide opportunity. So don’t be afraid to specialise.
So long as you can get a reasonable-sized bet down, limiting your focus to a few markets can reap great rewards. Specialising is often the path to long-term betting.
#5 Apply Sound Money Management
The key to betting on any sport is in applying a sound money management strategy. This primarily involves applying a consistent staking strategy, a strategy that will make the most of the value you have identified while suggesting a stake size that will protect your betting bankroll.
If you take your betting seriously, then you need to be serious about how you manage your money. Randomly betting a tenner here or a fiver there is only going to lose you money in the long run. To be a successful football bettor you need to approach your staking like a financial planner.
The saying ‘Only bet what you can afford to lose’ is as true as ever and any money management strategy should take this very seriously. Begin with a sum of money that you would be comfortable losing. Of course, we are aiming to make money betting on football. But even in possession of the sharpest football betting strategy, things can and will go wrong from time to time. Only through the application of a proven staking plan and money management strategy can you endure the worst of losing streaks and exploit the greatest of winning streaks.
To learn more, please read our guide to bankroll management as well as our guide to calculating the perfect bet stake using the Kelly Criterion.
#6 Keep A Record Of Your Football Bets
If you’re serious about betting on football, then you must, we repeat, must, keep a record of your betting. This record should include the following:
- Date, League/Tournament, Bet Type, Bet Details, Bookmaker, Your Stake, Your Odds, Profit/Loss, Comments
If you’re not keeping such records, then you are doomed to failure. In keeping a detailed record of your football betting, you will be able to review your results. You may sometimes say to yourself “I never win football bets”. If you do find yourself saying this, firstly, stop feeling sorry for yourself and secondly, in keeping detailed records, you’ll see that you are actually winning football bets at a higher rate than you assume. As bettors we can often have a tendency to recall losses more easily than we can our wins. But by keeping records of your bets, you’ll be able to see which leagues you’re making money on, the leagues that are diminishing your returns, not to mention the bet types you are most suited to and those markets that are consistently losing you money.
#7 Assess Performance Potential Accurately
Pundits like to talk about form. ‘This team is in great form’. ‘That team’s form has been terrible.’ You’ve not doubt heard it many times.
But what is problematic about ‘form’ is that it is fleeting. Just because a team has been in great form, is no indication that this form will continue or even that the ‘form’ was based on anything other than perhaps a run fixtures against lowly clubs, or more allusive yet, a run of good fortune.
When it comes to football betting, what we are really interested in is not so much whether or not a team is in form, but rather, what is their performance potential heading into a given match. There are many things to consider in this assessment:
Home vs Away performance
It’s no secret that on average, clubs perform better at home than away. In fact it has been shown that an average team is roughly a +0.74 goals better at home than they are away.
Despite this, the subject of home vs away performance is a hotly debated issue. While some clubs may display a distinct ability to perform better at home than away over a given time frame, the reasons for this may have little to do with home ground advantage. Some analysts believe that what may often appear to be a distinct home field advantage, may merely be a run of better than expected performance.
When you’re assessing a team’s potential to perform in a certain environment, be wary of falling prey to glib cliches such as ‘Oh this team is always tough at home’. The reality is that every team is better on their field than others, but the belief that certain clubs hold a distinct home field advantage that exceeds the average is often misleading.
Injuries & Suspensions
It’s obvious that we should consider both injuries and suspensions when assessing a team’s potential. But many people misjudge the affects of such absences. When it comes to both injuries and suspensions, what is key isn’t so much that a team will be missing a player, but who will replace that player. Is the replacement a significant downgrade? Or is the missing player easily replaced in the lineup?
This is where a deep knowledge of each team’s squad comes into play. Yes, many may know of the injured centre back but how many bettors know the performance potential of his lesser known replacement?
Further to this, how many bettors know how such an absence will impact a team’s tactical approach? In both regards, a thorough understanding of a team’s squad can profoundly assist us in the project of value football betting. Betting markets can often over estimate the impact of a missing star player as opposed to the absence of a lesser known but durable and consistent performer.
Fixture Demands
As we get deeper into the football calendar, the demands of fixture commitments come into play. This is definitely a key area of consideration for all football bettors. And just as we discussed previously in terms of injuries and suspensions, developing an intimate knowledge of each club’s squad will often help you spy value betting opportunities.
A Premier League club may for instance have a run of league commitments, with both Cup and European duties in between. What fixtures are they likely to take more seriously than others? How may they alternate their line up and how may a distinctly alternate lineup perform, both in terms of tactical approach and team chemistry? The ability to answer these questions accurately will more often than not provide you with value betting opportunities.
Tactical & Personnel Match-ups
There’s a saying in boxing that styles make fights. And this is also true in football. We will often see teams that we consider to be of inferior quality take it right up to more highly ranked opponents, often claiming unlikely victories or at the very least, causing headaches for their more fancied opponents.
When it comes to football betting, what we want to discern is whether or not such performances were the result of the inferior team providing a difficult tactical match up for their opponents in terms of personnel, whether such results were merely a matter of the superior team perhaps being in the midst of a congested schedule or whether or not such performances were a matter of random good fortune.
To evaluate these performances accurately we must not only be familiar with each club’s squad, but likewise be familiar with how each club likes to set up tactically and how a team’s personnel may impede their gameplan. If we can do this, and come to an accurate assessment of which inferior teams match up well with their more highly regarded opponents, betting value can easily be found.
Develop A Predictive Football Betting Model
Many successful football bettors have made their living through shrewd statistical analysis and mathematical modelling. What is involved in developing a football betting model? There is no single answer to this other than to say, whatever helps you identify football values bets. Some predictive football models are quite simple and only involve the use of goals scored for and against over a given sample size of matches played, while more sophisticated models incorporate a diverse range of statistical categories such as:
- Goals For/Against
- Shots For/Against
- Shots On Target For/Against
- Shot Location Goal Expectancy
- Possession Share
- Individual Player Performance
Regardless of what football statistics you choose to develop your model, the aim is the same – to assess the probability of a given match (or betting market) outcome more accurately than the bookmakers do.
The purpose of your football betting model is to identify betting value. It does not need to identify value in every match played or every betting market available. In fact, it’s likely your model may only identify one value bet each week of the Premier League season. That amounts to 38 bets per season.
Not many right?
But think if you could expand your model to cover 40 different football leagues. You’re then looking at a total of over 1,000 bets per season. The work involved in developing and maintaining a cunning betting model can be demanding, but the rewards can be immense.
#8 Exploit Market Biases By Betting Against The Crowd
Consistently betting on value opportunities is what will make you a successful football bettor in the long term. Yes, a team may be at long odds and yes, we still believe that the chances of them winning the match, while greater than what the bookmakers are suggesting, are still unlikely, but it is in repeatedly betting on situations such as these that will see you succeed betting on football in the long term.
When it comes to football betting there are two clear market biases:
- Short Priced Home Favourites
- Higher Than Average Goal Totals
Underdogs are typically better value than favourites, particularly home favourites. Why? Because most people betting on football are thinking short-term. They want a winner today. Not next week. Not next month. They want a “sure thing” now. So they place large bets on short-priced home favourites. This excessive betting money on short-priced favourites often distorts betting markets, which in turn artificially inflates the odds for underdogs, making such underdogs great value.
Let’s consider an example, England are playing a World Cup qualifier at home to San Marino. England, with their array of star players, are massive favourites and are priced at 1.10 to win. San Marino, being only semi-professional footballers and very rarely beating anybody, are priced at odds of 21.00. Who in their right mind would bet on San Marino to win? Well yes, it’s extremely unlikely that they will. The odds of 21.00 suggest that San Marino are roughly a 4.8% chance to win the match.
But through our research and analysis, we believe San Marino are closer to an 8% chance. Yes, even at 8% a San Marino win is still very unlikely. But we still believe the chances of them winning are greater than what the odds of 21.00 would suggest. Based on our analysis and our assessed probability of 8%, we believe the odds should be closer to 12.50. However unlikely that it is to be a winner, a bet on San Marino is a value opportunity and we should take it.
Another market bias is for higher than average goal totals. Why? Because the average football punter subconsciously bets with aggression. In this sense, betting on a low scoring match is a somewhat passive act and doesn’t satisfy this subconscious aspect of placing a bet. Add to this the fact that very few people want to watch a football match with a limited number of goals scored, and you get a market bias for higher than average goals. This bias artificially places downward pressure on the odds for markets such as Over 2.5 goals, leaving the Under 2.5 goals market to be the better value option more often than not.
Simply betting on both away underdogs and low goal totals won’t make you rich any time soon. However, they both present examples of why it is often better to bet against the crowd.
#9 Follow Football Betting Tips
Following football betting tips can be great especially if you can find a genuine expert in a league you may not normally bet on.
But let’s be clear. You should not follow any football tipster, expert or pundit blindly. There are literally hundreds of so-called expert football tipsters on the internet. Some even charge high subscription fees for the privilege of following their service. Some are legitimate, but most are not and will not only see you lose money through following their tips, but likewise waste money through their excessive subscription fees. There are also services claiming to have inside information on fixed matches, willing to sell that information for a price. Don’t be fooled by their claims.
Before you follow any football tipster, you should do your research. For example, there are hundreds of football tipsters at bettingexpert. And while each is sincere in their attempts to succeed from their football tips, the majority do not. There are however a select few who are reliable, often due to an intimate knowledge of a particular football league.
To learn more, please read our guide to following betting tips.
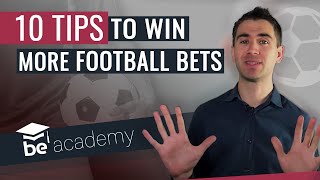
#10 Have Accounts With Multiple Bookmakers
Many football bettors limit their chances of success by limiting their ability to take the best odds available. Yes, there is a certain simplicity with using just one bookmaker for all your betting. But the reality is, if you want to bet on football long term, you need a number of bookmakers for your football betting.
Why? Because in limiting your bookmaker portfolio, you’re restricting yourself in terms of the odds available. For example, take a look at odds comparison site Smartbets. Below are the Asian Handicap odds for an upcoming match between Tottenham and Atletico Madrid.
This is but a simple example, but we can see that by merely having two competing bookmakers at our disposal, we can take the better of the odds available. By continually taking the best odds available among competing bookmakers, we severely increase our chances of betting on football long term.
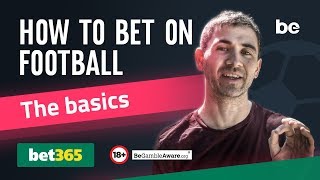